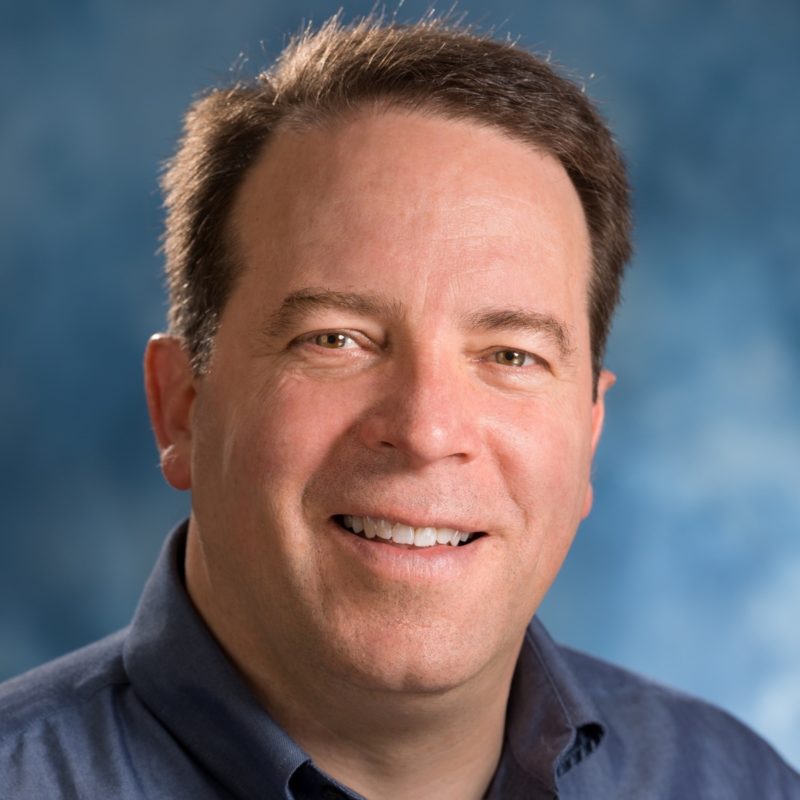
Speakers:
Against All Odds: The Slow, Startling Triumph of Reverend Bayes
Date:
Wednesday, Oct 16, 2019
Time:
9:15 am
Room:
Premium 2
Summary:
The core Bayesian idea, when learning from data, is to inject information — however slight — from outside the data. In real-world applications, meta-information is clearly needed — such as domain knowledge about the problem being addressed, what to optimize, what variables mean, their valid ranges, etc. But even when estimating basic features (such as rates of rare events), even vague prior information can be very valuable. This key idea has been re-discovered in many fields, from the James-Stein estimator in mathematics and Ridge or Lasso Regression in machine learning, to Shrinkage in bio-statistics and “Optimal Brain Surgery” in neural networks. It’s so effective — as I’ll illustrate for a simple technique useful for wide data, such as in text mining — that the Bayesian tribe has grown from being the oppressed minority to where we just may all be Bayesians now.